PROGRAM
The final conference program is currently publicly accessible here.
Plenary lectures
NOLCOS 2025 features the following plenary speakers :
- Francesco Bullo (University of California, Santa Barbara)
- Moritz Diehl (University of Freiburg, Germany)
- Maurice Heemels (Eindhoven University of Technology (TU/e))
- Sophie Tarbouriech (LAAS-CNRS, France)
More details about the dates and contents of the plenary lectures are provided below.
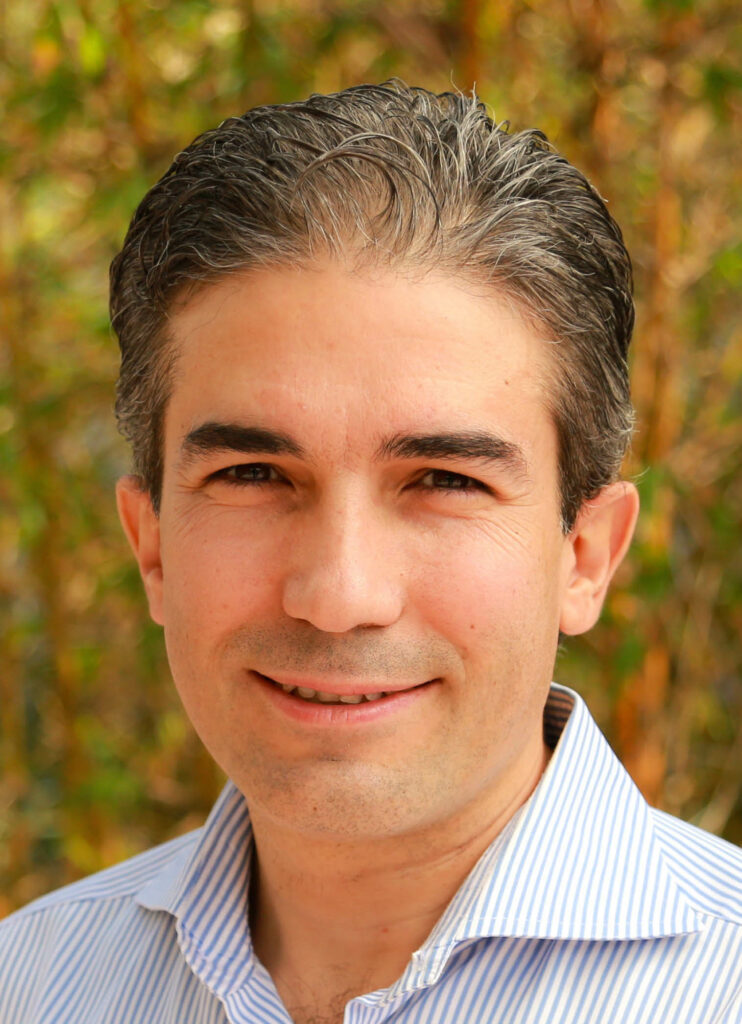
Francesco Bullo
Title : Contraction Theory for Optimization, Control, and Neural Networks
Session : To be defined
University of California, Santa Barbara
Bio : Francesco Bullo is a Distinguished Professor of Mechanical Engineering at the University of California, Santa Barbara, CA, USA. He was previously with the University of Padova (Laurea degree, 1994), Italy, the California Institute of Technology (Ph.D. degree, 1998), Pasadena, CA, and the University of Illinois at Urbana Champaign, IL, USA. His research interests include contraction theory, network systems, and distributed control. He is the author or coauthor of Geometric Control of Mechanical Systems (Springer, 2004), Distributed Control of Robotic Networks (Princeton, 2009), Lectures on Network Systems (KDP, 2024), and Contraction Theory for Dynamical Systems (KDP, 2024). He served as IEEE CSS President and SIAG CST Chair. He is a Fellow of ASME, IEEE, IFAC, and SIAM.
Abstract : This talk surveys recent advances on contraction theory for dynamical systems, as a robust, computationally-friendly and modular stability theory. Starting from basic notions, I will present novel theoretical properties and examples of contracting dynamics, including gradient systems, controlled Lure’ systems, constrained optimization solvers, and multiplayer games. As first application I will discuss online feedback optimization, where a dynamic plant is interconnected with a controller based on first-order optimization methods. Second, I will discuss the contractivity properties of recurrent neural networks and briefly review applications to unsupervised representation learning, implicit learning models, and reservoir computing.
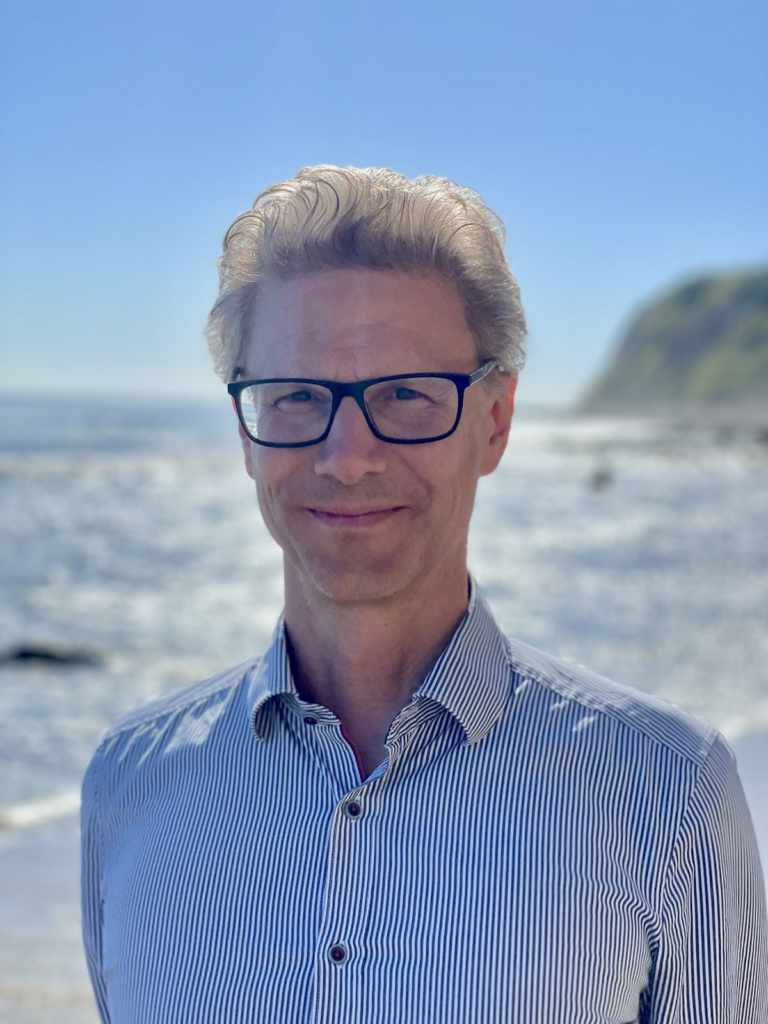
Moritz Diehl
Title : Bayesian optimization for optimal control problems with outer structure and its application to iterative learning control
Session : To be defined
University of Freiburg, Germany
Bio : Moritz Diehl currently heads the Systems Control and Optimization Laboratory at the University of Freiburg, Germany. He was born in Hamburg in 1971 and studied physics and mathematics in Heidelberg and Cambridge University from 1993-1999. He received his Ph.D. in 2001 from Heidelberg University, at the Interdisciplinary Center for Scientific Computing. From 2006 to 2013, he was a professor with the Department of Electrical Engineering, KU Leuven University Belgium, and served as the Principal Investigator of KU Leuven’s Optimization in Engineering Center OPTEC. Since 2013, he is at the University of Freiburg, where he is affiliated to the Department of Microsystems Engineering (IMTEK) and co-affiliated to the Department of Mathematics. Among other, he has served as associate editor of Journal of Optimization Theory and Applications (JOTA), Optimal Control Applications and Method (OCAM), and International Journal of Control, and was assembly and council member of the European Control Association (EUCA) from 2013-2019. He has co-organized several workshops and conferences, e.g. the 14th Belgian-French-German Conference on Optimization 2009 in Leuven, Belgium, the 7th International Airborne Wind Energy Conference 2017 in Freiburg, Germany, the IPAM Workshop on Intersections between Control, Learning and Optimization in 2020 at the Institute for Pure and Applied Mathematics, University of California, Los Angeles, US, and the IFAC Conference on Nonlinear Model Predictive Control NMPC 2024 in Kyoto, Japan. At the University of Freiburg, he served as dean of studies of the Faculty of Engineering in 2018-2020, and since 2023 he is the managing director of Freiburg University’s Center for Renewable Energy (ZEE). His research interests are in optimization and control, spanning from numerical method and software development to applications in different branches of engineering, with a focus on embedded systems and on renewable energy systems.
Abstract : In this talk we consider Bayesian optimization (BO) for problems with known outer problem structure. In contrast to the classic BO setting, where the objective function itself is unknown and needs to be iteratively estimated from noisy observations, we analyze the case where the objective has a known outer structure – given in terms of a loss function – while the inner structure – an unknown input-output model – is again iteratively estimated from noisy observations of the model outputs. We introduce a novel lower confidence bound algorithm for this particular problem class which exploits the known outer problem structure. Numerical simulations illustrate the performance of the proposed method in comparison to both structure-exploiting and structure-agnostic approaches. The talk presents joint work with Katrin Baumgärtner.
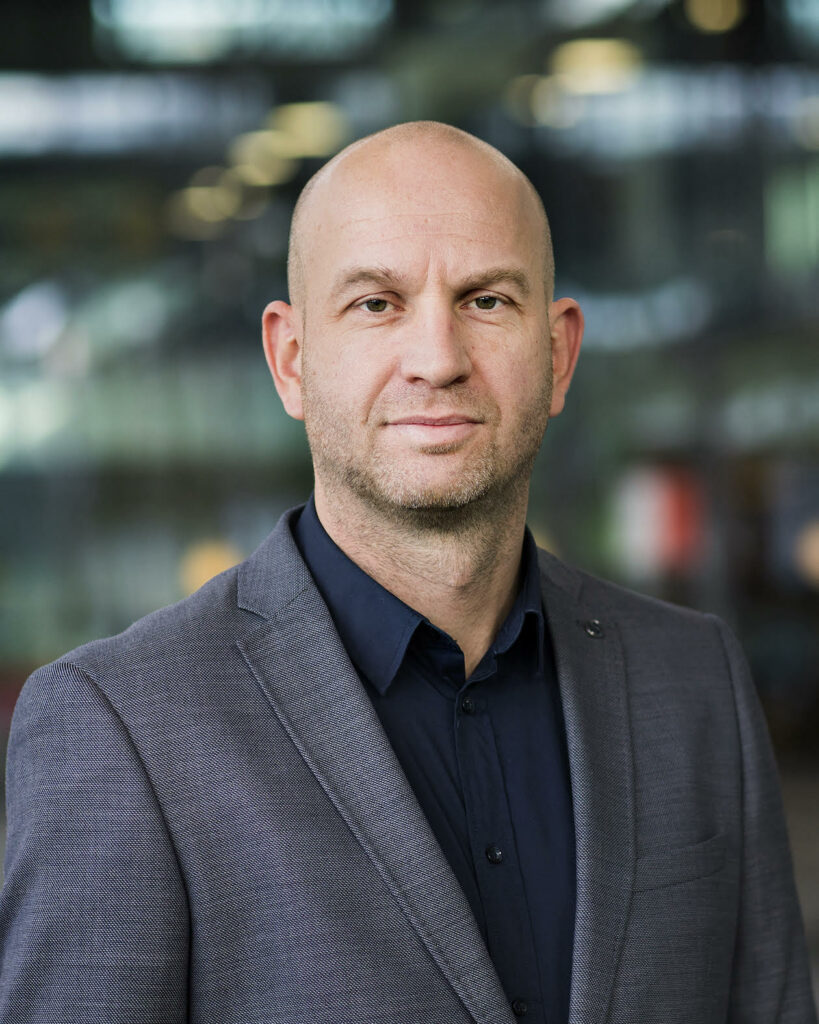
Maurice Heemels
Title : Projected Dynamics in Control
Session : To be defined
Eindhoven University of Technology (TU/e)
Bio : Maurice Heemels received M.Sc. (mathematics) and Ph.D. (control theory) degrees (summa cum laude) from the Eindhoven University of Technology (TU/e) in 1995 and 1999, respectively. From 2000 to 2004, he was with the Electrical Engineering Department, TU/e, as an assistant professor, and from 2004 to 2006 with the Embedded Systems Institute (TNO-ESI) as a research fellow. Since 2006, he has been with the Department of Mechanical Engineering, TU/e, where he is currently a Full Professor and Vice-Dean. Maurice held visiting professor positions at Swiss Federal Institute of Technology (ETH), Switzerland (2001), University of California at Santa Barbara (2008) and University of Lorraine, France (2020). His current research includes hybrid and cyber-physical systems, networked and event-triggered control systems and model predictive control. Dr. Heemels served/s on the editorial boards of Automatica, Nonlinear Analysis: Hybrid Systems, Annual Reviews in Control, and IEEE Transactions on Automatic Control. He was a recipient of a personal VICI grant awarded by NWO (Dutch Research Council) and an ERC Advanced Grant (European Research Council). He is a Fellow of the IEEE and IFAC. He was the recipient of the 2019 IEEE L-CSS Outstanding Paper Award and the 2020 Automatica Paper Prize Award. He was in the IEEE-CSS Board of Governors (2021-2023) and the chair of the IFAC Technical Committee on Networked Systems (2017-2023). He is the Editor-in-Chief of the IFAC journal Nonlinear Analysis: Hybrid Systems since 2023. He is happily married and proud father of two kids.
Abstract : Projected Dynamical Systems (PDSs) are a class of discontinuous dynamical systems, stemming from economics, in which projection of dynamics is used to keep the state of the system in specific constraint sets. In this talk we will focus on the opportunities that PDS may offer for designing high-performance nonlinear control systems. The key idea is to add projection operators to the controller dynamics to constrain the controller’s input-output (i/o) behavior to tailored sets, directly enhancing overall performance. As a prototypical projected controller, we will discuss the Hybrid Integrator-Gain System (HIGS) being a projected integrator that ensures the sign equivalence of its input and output, thereby avoiding the 90-degree phase lag inherent to linear integrators. In this way, projected controllers such as HIGS can overcome well-known fundamental performance limitations related to linear time-invariant (LTI) control. The study of HIGS and its generalizations calls for an extension of classical PDS, called “extended PDS (ePDS)”, as only partial projection of the closed-loop states is allowed; we can only change the controller states by projection, not the plant states. For the class of projected controllers (and their formalizations in terms of ePDSs), we will discuss existence and forward completeness of solutions, their sampled-data implementations, and input-to-state and incremental stability properties. Both Lyapunov-based and frequency-domain conditions will be provided. Incremental stability properties will be used to guarantee unique steady-state responses to external excitation and will serve as a foundation for developing “nonlinear Bode plots” for accurate closed-loop performance evaluation. Moreover, connections will be made to dynamics based on Control Barrier Functions (CBFs). Finally, we will demonstrate the design and implementation of projected controllers through experiments on advanced motion systems, showcasing their practical potential in industry.
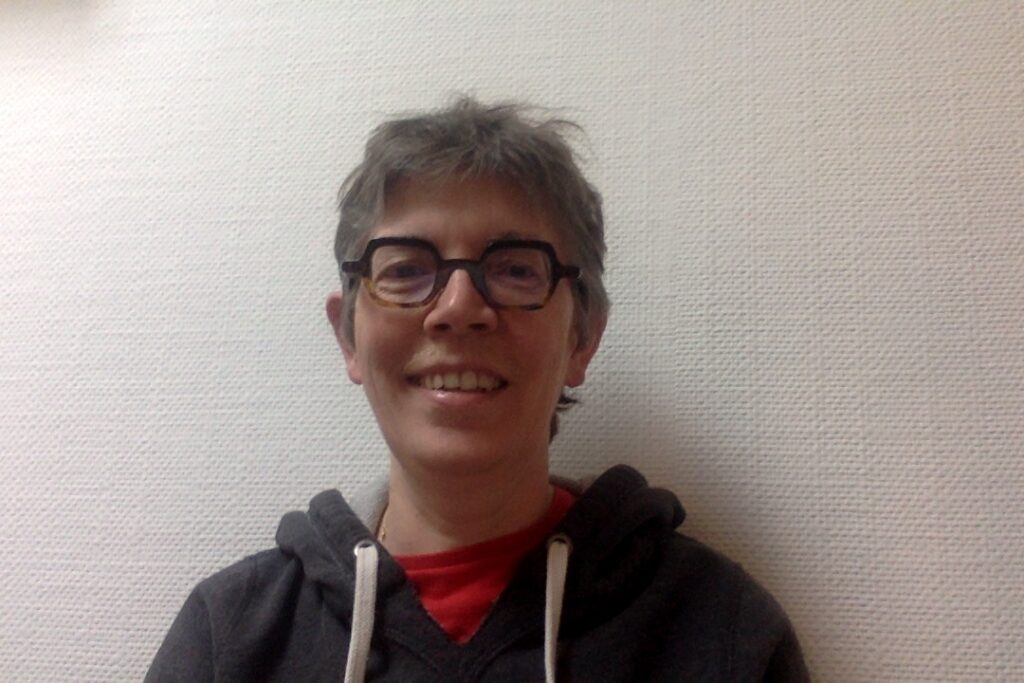
Sophie Tarbouriech
Title : Sign-indefinite quadratic Lyapunov functions for control
Session : To be defined
LAAS-CNRS, France
Bio : Sophie Tarbouriech received the Ph.D. degree in Control Theory in 1991 and the HDR degree (Habilitation à Diriger des Recherches) in 1998 from University Paul Sabatier, Toulouse, France. Currently, she is a Senior Researcher at CNRS and a member of LAAS- CNRS, Toulouse. Her main research interests include analysis and control of linear and nonlinear systems with constraints (limited information), hybrid dynamical systems. She is currently Associate Editor for SIAM Journal of Control and Optimization and Senior Editor for Automatica. She is also Deputy Editor-in-Chief of Automatica. She is a member of IFAC and IEEE Technical Committees on Nonlinear Systems and Hybrid Systems. She is IEEE Fellow for contributions to nonlinear control systems with isolated nonlinear elements.
Abstract : In this talk, we will overview the use of a class of sign-indefinite quadratic Lyapunov functions comprising an extended quadratic form. We will first discuss stability/performance analysis results certified by these functions in linear saturated feedbacks, and their use for local or global anti-windup design. Second, their use for the direct design of linear plant-order saturated output-feedback controllers will be presented. Furthermore, we will then illustrate how the technique can be extended to deal with different nonlinearities and to handle other kinds of problems as for example the convergence of some optimization algorithms or the contraction problem.
Semi-plenary lectures
NOLCOS 2025 features the following semi-plenary speakers :
- Peter Giesl (University of Sussex, UK)
- Simona Onori (Stanford University, California)
- Ian Manchester (University of Sydney, Australia)
- Ricardo Sanfelice (University of California Santa Cruz)
More details about the dates and contents of the semi-plenary lectures are provided below.
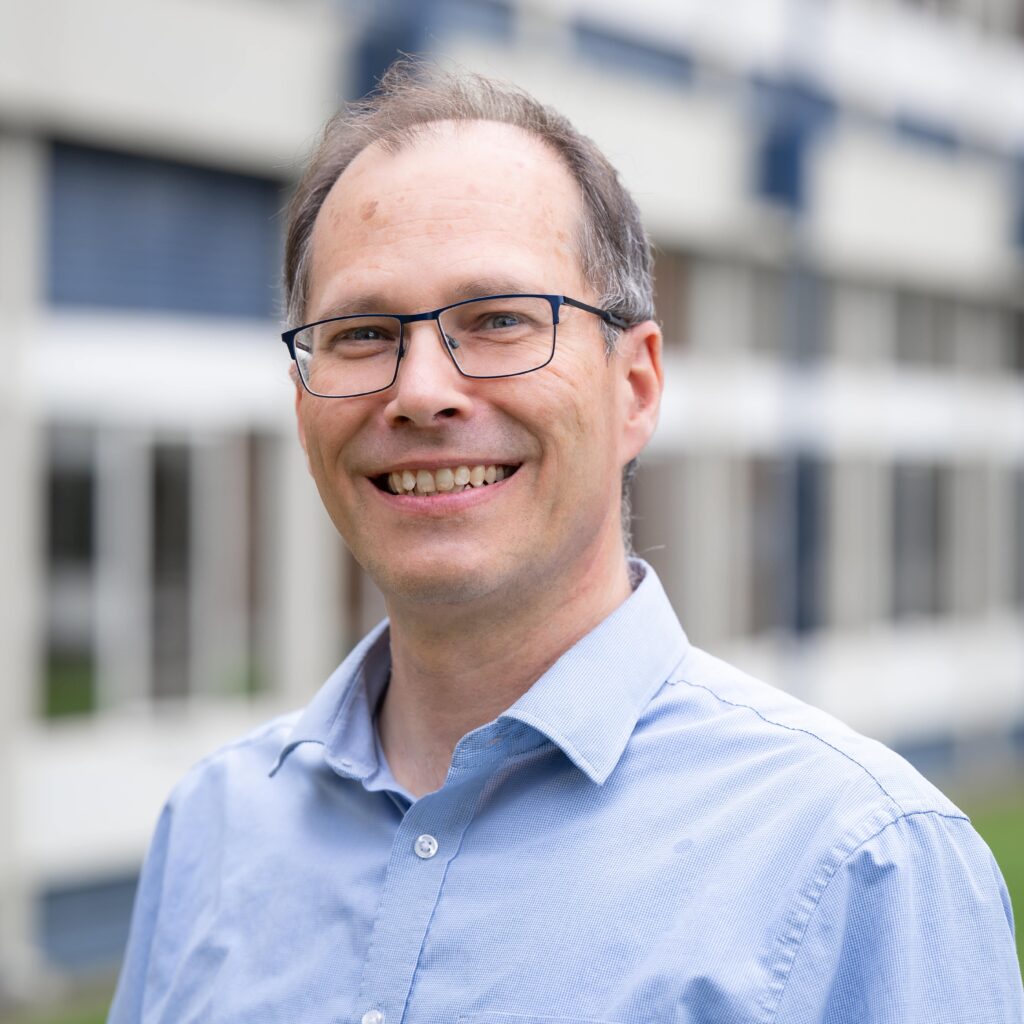
Peter Giesl
Title : Numerical determination of basins of attraction for dynamical systems
Session : To be defined
University of Sussex, UK
Bio : Peter Giesl studied Mathematics in Hamburg (Germany) and Paris (France); he obtained a PhD and Habilitation in Mathematics from the TU Munich (Germany). After a year as Acting Professor in Augsburg (Germany), he became Lecturer and later Professor at the University of Sussex (UK), where he is currently Head of Department of Mathematics. His research interests are in analytical and numerical aspects of dynamical systems, in particular the determination of attractors and their basins of attraction. He has written over 100 research papers, including reviews on computational methods for Lyapunov functions and contraction metrics.
Abstract : We consider a general dynamical system, either continuous-time, given by solutions of an ordinary differential equation, or discrete-time, given by the iteration of a map. The long-term behaviour can be characterised by attractors and their corresponding basins of attraction. Tools to determine the basin of attraction include (complete) Lyapunov functions and contraction metrics. A complete Lyapunov function is a scalar-valued function which decreases along solutions; attractors are local minima and their basin of attraction can be determined using sublevel sets. A contraction metric is a metric such that the distance between adjacent solutions decreases with respect to the metric, and thus they share the same long-term behaviour. In this talk I will discuss the numerical construction of (complete) Lyapunov functions and contraction metrics to determine the basins of attraction of a given system. In particular, I will present computational methods using meshfree collocation with Radial Basis Functions (RBF) as well as Continuous Piecewise Affine (CPA) functions, compare them and illustrate them with examples.
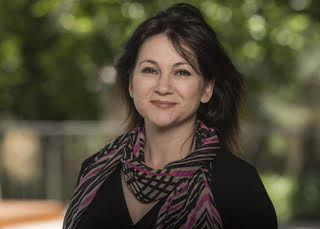
Simona Onori
Title : The Role of Control in the clean energy revolution: the Lithium-Ion battery case study
Session : To be defined
Stanford University, California
Bio : Simona Onori is an Associate Professor in Energy Science and Engineering at Stanford University, where she also holds a courtesy appointment in Electrical Engineering. She directs the Stanford Energy Control Lab and is a Senior Fellow at the Precourt Institute for Energy at Stanford. Onori is an SAE Fellow and serves as the Editor-in-Chief of the SAE International Journal of Electrified Vehicles since 2020. She was a Distinguished Lecturer for the IEEE Vehicular Technology Society from 2020 to 2022 and has been a Senior Member of IEEE since 2015. Her research focuses on the modeling, control, and estimation of energy systems, with particular focus to hybrid electric vehicles and electrochemical processes, such as battery technologies. She earned a Laurea Degree in Computer Science from University of Rome “Tor Vergata”, an M.S. in Electrical Engineering from University of New Mexico, and a PhD. in Control Engineering from University of Rome “Tor Vergata”. https://onorilab.stanford.edu/simona-onori
Abstract : Lithium-ion batteries play a critical role in the clean energy transition, powering electric vehicles (EVs) and enabling renewable energy storage systems. Once deployed, these batteries encounter significant challenges, including the difficulty of accurately estimating state-of-charge (SoC) and state-of-health (SoH), which impact their long-term performance, safety, and reliability. This semi-plenary explores innovative solutions to these challenges through the integration of advanced modeling, control strategies, real-world data and laboratory aging campaigns. We begin by introducing the physics underlying lithium-ion battery operation and the role of electrochemical models in understanding battery behavior. To address the lack of direct measurements, we propose an adaptive, electrode-based, interconnected observer based on the enhanced single-particle electrochemical model for real-time SoH estimation. This observer captures aging mechanisms such as solid electrolyte interphase growth, enabling accurate estimation of lithium concentration, capacity, and aging-sensitive parameters. Hardware-in-the-loop validation of the system will also be presented. We then present findings from laboratory aging campaigns comparing dynamic discharge profiles, which simulate real-world EV driving conditions, to constant-current profiles. Dynamic profiles enhance battery longevity, with explainable machine learning revealing the critical role of low-frequency current pulses and time-induced aging in degradation mechanisms. This emphasizes the need for realistic load profiles in battery design and testing. Additionally, field data from EV operation will be discussed which highlight misalignments between laboratory testing and real-world usage, underscoring the importance of refining testing protocols. Finally, we address the challenges of deploying retired EV batteries in second-life applications like grid-scale energy storage. An online adaptive health estimation method is proposed, leveraging real-time operational data to enable SoH monitoring without requiring historical usage data.
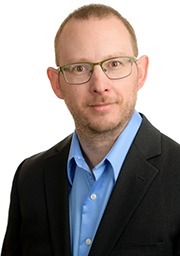
Ian Manchester
Title : Neural Networks in the Loop: Learning with Control-Theoretic Guarantees
Session : To be defined
University of Sydney, Australia
Bio : Ian R. Manchester received the B.E. (Hons 1) and Ph.D. degrees in electrical engineering from the University of New South Wales, Sydney, NSW, Australia, in 2002 and 2006, respectively. From 2006-2009 he was a post-doctoral researcher at Umea University, Sweden, and from 2009-2012 he was a Research scientist at the Massachusetts Institute of Technology. Since 2012 he has been a faculty member at the University of Sydney, Australia, where he is currently Professor of Mechatronic Engineering, Director of the Australian Centre for Robotics, and Director of the Australian Robotic Inspection & Asset Management Hub. His current research focuses on algorithms for control, estimation, and learning of nonlinear dynamical systems, with applications in robotics, robust machine learning, and other fields.
Abstract : Neural networks trained on large data sets continue to astonish with their capacity to learn highly sophisticated functions and diverse behaviours, raising hopes for a pathway to vastly more “intelligent” feedback policies in robotics and cyber-physical systems. However this potential seems to come through a Faustian bargain in which we sacrifice all guarantees about robustness, stability, safety, and performance. In this talk, we will show that this need not be the case. We will describe a new approach to parameterizing neural networks models, both static (feed-forward) and dynamic (recurrent), with built-in guarantees relevant to control applications. We will trace the connections between convex parameterizations from robust and nonlinear control to so-called “direct” parameterizations, i.e. smooth & unconstrained parameterizations of neural networks satisfying prescribed behavioural constraints. These parameterizations enable learning of provably robust models and policies via simple first-order methods, without any auxiliary constraints or projections. We will illustrate the approach through applications in certifiably-robust machine learning, learning of Lyapunov functions and stable dynamical systems, physics-informed learning of nonlinear observers, parameterization of robust nonlinear controllers, and learning of ‘easy-to-optimize’ surrogate loss functions.
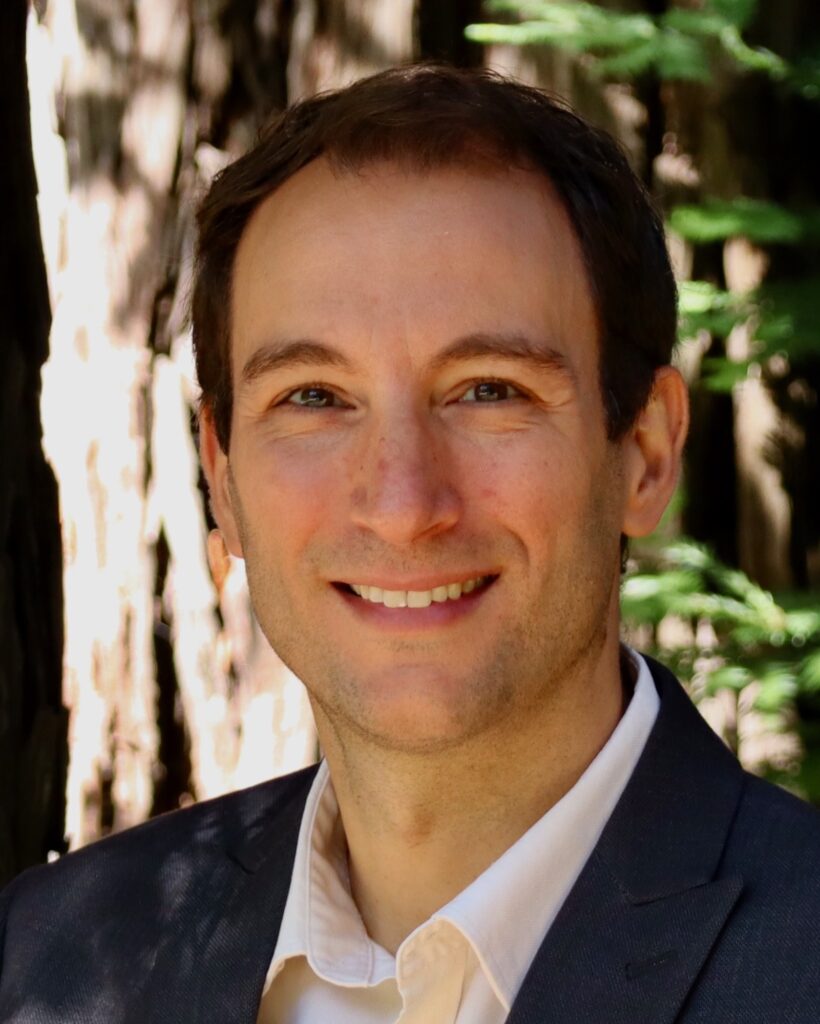
Ricardo Sanfelice
Title : Advancing Control Theory with Hybrid Dynamics: From Stability and Optimization to AI-driven Control
Session : To be defined
University of California, Santa Cruz
Bio : Ricardo G. Sanfelice is Professor and Department Chair of Electrical and Computer Engineering, University of California at Santa Cruz. He received his M.S. and Ph.D. degrees in 2004 and 2007, respectively, from the University of California, Santa Barbara. During 2007 and 2008, he was a Postdoctoral Associate at the Laboratory for Information and Decision Systems at the Massachusetts Institute of Technology and visited the Centre Automatique et Systemes at the Ecole de Mines de Paris for four months. Prof. Sanfelice is the recipient of the 2013 SIAM Control and Systems Theory Prize, the National Science Foundation CAREER award, the Air Force Young Investigator Research Award, the 2010 IEEE Control Systems Magazine Outstanding Paper Award, the 2012 STAR Higher Education Award for his contributions to STEM education, and the 2020 ACM Test-of-Time Award from the HSCC. He is Associate Editor for Automatica, Communicating Editor for the Journal of Nonlinear Science, Springer, a Fellow of the IEEE, and served as Chair of the Hybrid Systems Technical Committee from the IEEE Control Systems Society. He coauthored articles selected as finalists for the Best Student Paper Award (2014, 2019, and 2022) at the American Control Conference (ACC) and the International Conference on Automation Science and Engineering (CASE). He is Director of the Cyber-Physical Systems Research Center at UCSC and Director of the Center for Information Technology Research in the Interest of Society and the Banatao Institute (CITRIS) Aviation Initiative. His research interests are in modeling, stability, robust control, observer design, and simulation of nonlinear and hybrid systems with applications to robotics, power systems, aerospace, and biology.
Abstract : Hybrid dynamics are ubiquitous in most feedback systems, appearing in systems as diverse as autonomous vehicles, space robotics, biological networks, to just list a few. These systems pose significant challenges that classical control theory, despite its powerful methodologies, often fails to address. In fact, the presence of topological obstructions, limited and intermittently available information, and the intrinsic complexity of the dynamics hinder the certification of crucial properties such as stability, robustness, and safety. Compounding these challenges is the growing usage of computational control methods, such as reinforcement learning and large language model-based controllers, which often lack the provable guarantees or readily available certificates required for rigorous analysis. This talk will explore how hybrid systems and control extend the boundaries of traditional control theory, providing innovative tools capable of addressing problems that classical methods cannot solve, leading to advances in the context of emerging computational control methods and optimization.
Evening lecture
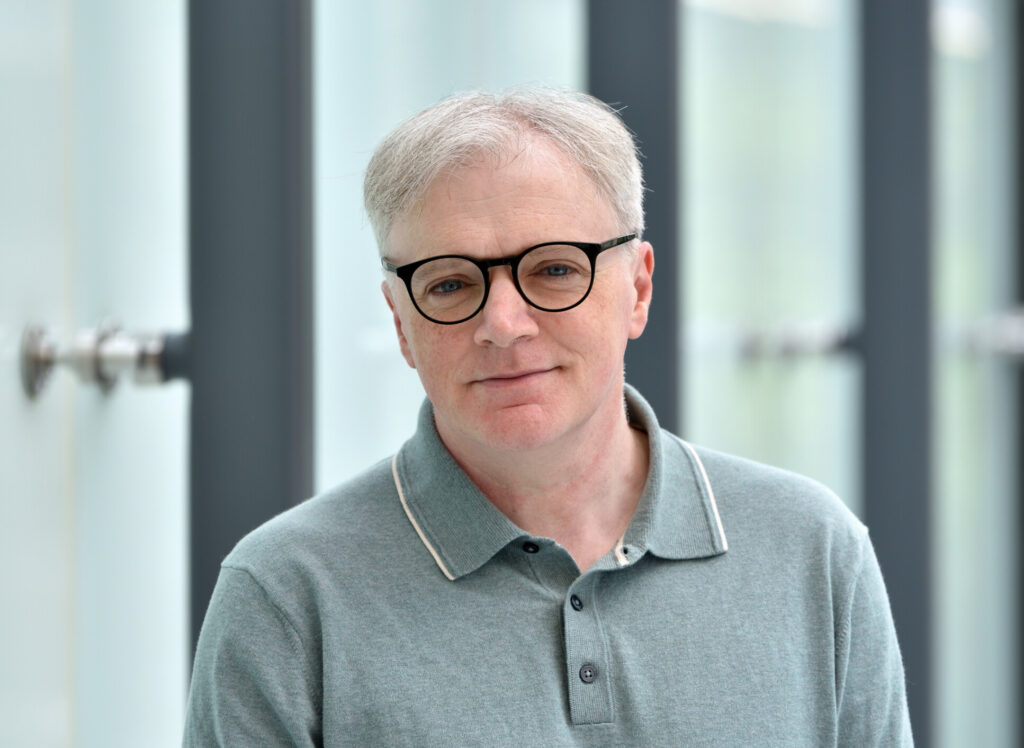
Robert N. Shorten
Title : Algorithmic Fairness by Design
Session : To be defined
Imperial College London, UK
Bio : Robert N. Shorten received his Ph.D. degree from University College Dublin, Ireland in 1997. From 1993 to 1997, he was employed, and the Holder of a Marie Curie Fellowship, with Daimler-Benz Berlin, Germany, where he conducted research in the area of smart gearbox systems. Following a brief period with the Centre for Systems Science, Yale University, where he worked with Prof. K. S. Narendra, he returned to Ireland as the holder of a European Presidency Fellowship in 1997. He co-founded of the Hamilton Institute in 2001 at the National University of Ireland Maynooth, Ireland, where he was a Full Professor until 2016. Professor Shorten has been a research visitor at Technion and Yale University, and a visiting
Professor at TU Berlin, Germany. From 2013-2016 he was seconded to IBM Research where he led the Optimization, Control and Decision Sciences team in the Smarter Cities Research Center. From 2016-2019 he was a Professor of Control and Decision Science at University College Dublin, and until 2019 held a part-time/consultancy role with IBM Research. Professor Shorten currently holds the Chair in Cyber-Physical Systems Design in the Dyson School of Design Engineering at Imperial College London. He is currently also Head of School at the Dyson School of Design Engineering, a founding Co-Director of the Centre for Sectoral Economic Growth at Imperial College London, and the academic lead (ICL) for the TU Munich-Imperial alliance. He is also Head of Research and Development of the electric mobility company Go Eve (www.goeve.com), the global co-lead for Climate and
Sustainability for the AI Alliance (https://thealliance.ai), and a former Chair of the Board of Directors at Future Mobility Campus Ireland.
Abstract : Algorithmic Fairness has recently become an issue of major societalprominence due (primarily) to the widespread deployment of AI assistant systems (see for example the AI Act introduced by European Commission https://artificialintelligenceact.eu). While it is certainly true that AI has acted as a catalyst for a large and emerging volume of research that is centered on detecting and mitigating bias in algorithmic systems, the issue of algorithmic fairness extends well beyond AI, and is in fact a major issue when designing any form of algorithmic system that seeks to enforce a social contract (systems that seek to assist and influence humans in sharing resources). While it is perhaps not well known, Control
and Systems Theory has made important contributions to this domain in the past, and is now well positioned to assist in the development of contemporary societal systems that are fair by design. Our objective in this talk is to introduce several problems where the issue of algorithmic fairness has emerged as a specific challenge in a Control and Systems Theory context. A specific focus of the talk will on problems where instability by design (in non-linear systems) is used a mechanism for fairness, on pricing algorithms, and on new directions of emergent research in the area of Optimal Transport (arising from the Autofair project). We shall conclude by presenting several open problems in this new and emerging domain.
Social Programme
Event: Welcome Reception
Date: Tuesday, 22 July
Time: 18:00~21:00
Location: Conference venue (Háskólatorg)
Event: Conference Banquet
Date: Thursday, 24 July
Time: 19:30~22:00
Location: Nauthóll, Nauthólsvegur 106, 101 Reykjavík (walking distance from the conference venue)
Event: Farewell Reception
Date: Friday, 25 July
Time: 13:00~15:00
Location: Conference venue (Háskólatorg)
Morning and afternoon coffee breaks, and lunches will be served at the conference venue