PROGRAM
Conference Program:
https://ifac.papercept.net/conferences/conferences/SYSID24/program/
A mobile-friendly version of the program is available on the IFAC app, which can be downloaded from the App Store and Good Play. Please log in using your IFAC affiliate credentials. For issues, email us at msznaier@coe.neu.edu (cc m.imani@northeastern.edu), and indicate which email you used in your IFAC affiliate account.
Program at a glance
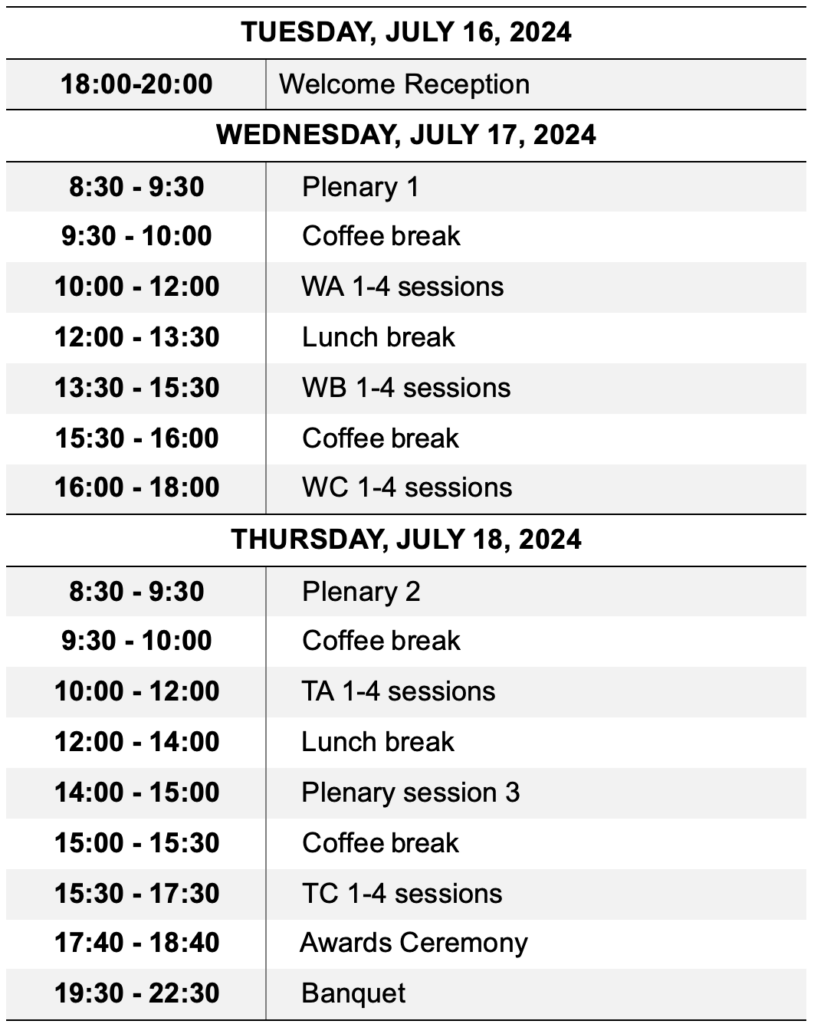
Plenary Talks
SYSID 2024 features the following plenary speakers :
Professor Roland Toth, Eindhoven University of Technology, The Netherlands, https://rolandtoth.eu
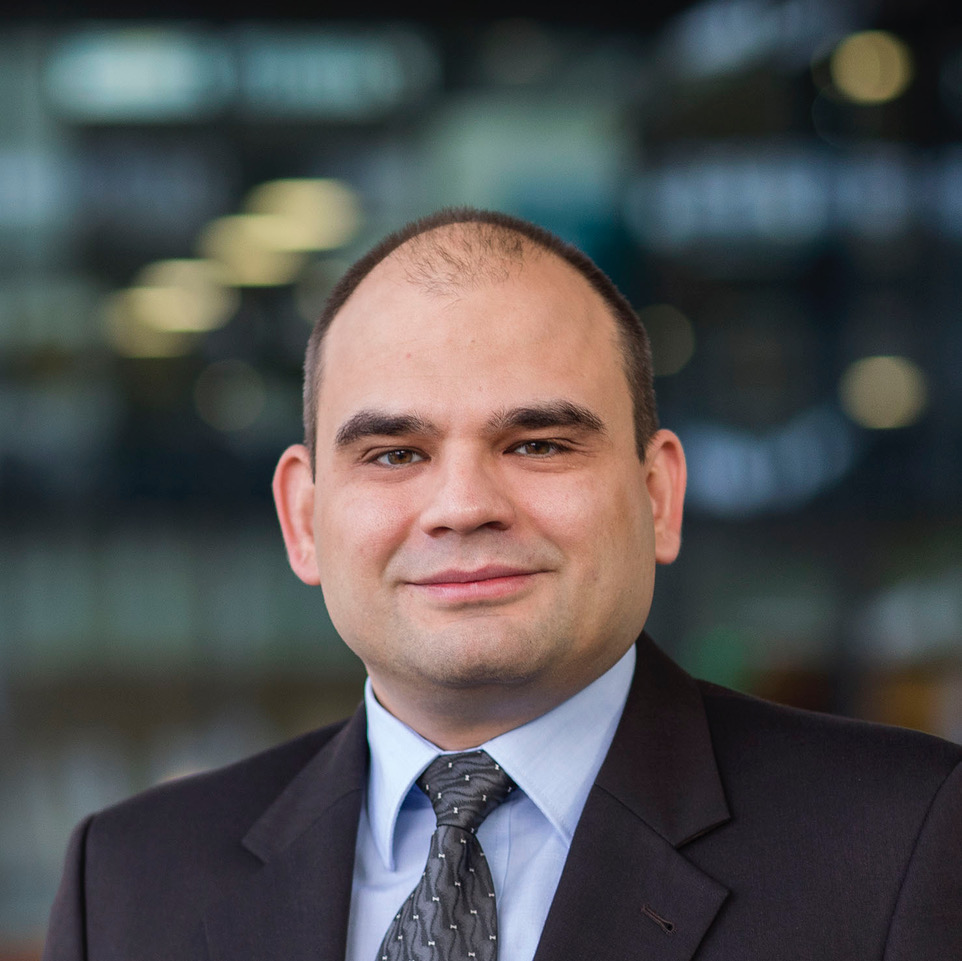
Title: Identification of Linear Parameter-Varying Models: Past, Present, and Beyond
Abstract
The framework of Linear Parameter Varying (LPV) systems has been established with the purpose to represent complex nonlinear and/or time-varying systems in terms of simple linear surrogate models that can easily be used for analyzing the system behavior or designing controllers. It has become a manifestation of a dream in engineering about the extension of powerful and well-understood analysis and design tools available for Linear Time Invariant (LTI) systems that allow regulation and shaping of the behavior of nonlinear/time-varying systems. The resulting LPV methods have provided stability and performance guarantees and contributed seriously to the advancement of aerospace engineering, automotive technologies, and the mechatronic industry over the years.
However, the question how such LPV models can be identified with the help of data instead of cumbersome model conversion tools gave rise to a serious research effort on the development of LPV system identification. This talk will overview the most advanced results of this scientific journey that spanned from prediction error minimization methods through subspace identification to learning-based methods using Reproducing Kernel Hilbert Space (RKHS) / Gaussian Process estimators and Deep Neural Networks (DNNs), capable for automated model structure selection and efficient choice of scheduling variables. We will showcase the methods on application examples and demonstrate the easiness to apply them in Matlab through the LPVcore toolbox.
Bio
Roland Tóth received his Ph.D. degree with Cum Laude distinction at the Delft University of Technology (TUDelft) in 2008. He was a post-doctoral researcher at TUDelft in 2009 and at the Berkeley Center for Control and Identification, University of California in 2010. He held a position at TUDelft in 2011-12, then he joined to the Control Systems (CS) Group at the Eindhoven University of Technology (TU/e). Currently, he is a Full Professor at the CS Group, TU/e and a Senior Researcher at the Systems and Control Laboratory, HUN-REN Institute for Computer Science and Control (SZTAKI) in Budapest, Hungary. He is Senior Editor of the IEEE Transactions on Control Systems Technology.
His research interests are in identification and control of linear parameter-varying (LPV) and nonlinear systems, developing data-driven and machine learning methods with performance and stability guarantees for modeling and control, model predictive control and behavioral system theory. On the application side, his research focuses on advancing reliability and performance of precision mechatronics and autonomous robots/vehicles with nonlinear, LPV and learning-based motion control.
He has received the TUDelft Young Researcher Fellowship Award in 2010, the VENI award of The Netherlands Organization for Scientific Research in 2011, the Starting Grant of the European Research Council in 2016 and the DCRG Fellowship of Mathworks in 2022. He and his research team has participated in several international (FP7, IT2-ESCEL, etc.) and national collaborative research grants.
Professor Sarah Dean, Cornell University, https://sdean.website/
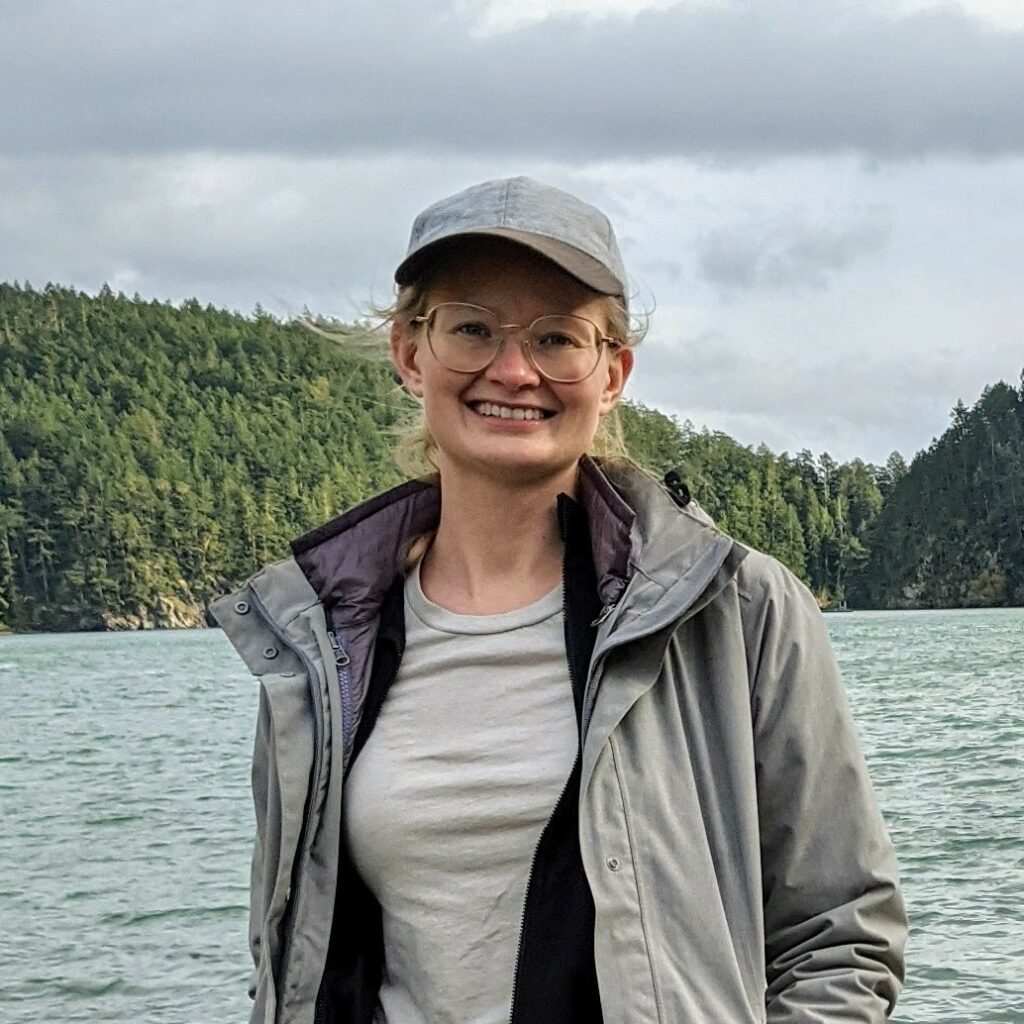
Title: Learning Models of Dynamical Systems from Finite Observations
Abstract
Despite the long history of the field of system identification, finite sample error guarantees have been developed only within the last decade. In this talk, I will discuss the finite sample perspective, including motivations and challenges. I will review the example of Linear Quadratic (LQ) control, where finite sample error guarantees have played an important role in the development and analysis of algorithms for adaptive and robust control. This line of work has strengthened connections between systems/control and computer science, particularly in the theory of reinforcement learning (RL). However, many motivating applications for RL, ranging from robotics to recommendation systems, exhibit phenomena not captured by the LQ example, like nonlinearities and partial observability. I will conclude by describing classes of dynamical systems which capture these challenges while maintaining theoretical tractability. I will describe some recent progress towards developing finite sample guarantees in these settings, and highlight the challenges that remain.
Bio
Sarah is an Assistant Professor in the Computer Science Department at Cornell. She is interested in the interplay between optimization, machine learning, and dynamics, and her research focuses on understanding the fundamentals of data-driven control and decision-making. This work is grounded in and inspired by applications ranging from robotics to recommendation systems. Prior to Cornell, Sarah earned a PhD in EECS from UC Berkeley and did a postdoc at the University of Washington.
Professor Rene Vidal, University of Pennsylvania, http://www.vision.jhu.edu/rvidal.html
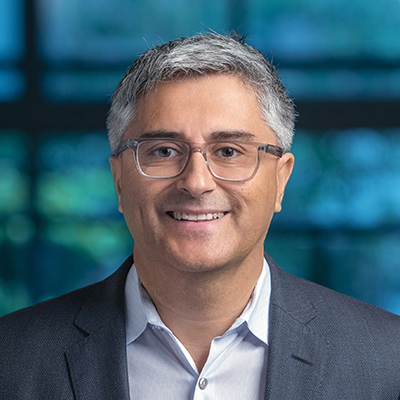
Title: Learning Dynamics of Overparametrized Neural Networks
Abstract
This talk will provide a detailed analysis of the dynamics of gradient based methods in overparameterized models. For linear networks, we show that the weights converge to an equilibrium at a linear rate that depends on the imbalance between input and output weights (which is fixed at initialization) and the margin of the initial solution. For ReLU networks, we show that the dynamics has a feature learning phase, where neurons collapse to one of the class centers, and a classifier learning phase, where the loss converges to zero at a rate 1/t.
Bio
René Vidal is the Penn Integrates Knowledge and Rachleff University Professor of Electrical and Systems Engineering & Radiology and the Director of the Center for Innovation in Data Engineering and Science (IDEAS) at the University of Pennsylvania. He is also an Amazon Scholar, an Affiliated Chief Scientist at NORCE, and a former Associate Editor in Chief of TPAMI. His current research focuses on the foundations of deep learning and trustworthy AI and its applications in computer vision and biomedical data science. His lab has made seminal contributions to motion segmentation, action recognition, subspace clustering, matrix factorization, global optimality in deep learning, interpretable AI, and biomedical image analysis. He is an ACM Fellow, AIMBE Fellow, IEEE Fellow, IAPR Fellow and Sloan Fellow, and has received numerous awards for his work, including the IEEE Edward J. McCluskey Technical Achievement Award, D’Alembert Faculty Award, J.K. Aggarwal Prize, ONR Young Investigator Award, NSF CAREER Award as well as best paper awards in machine learning, computer vision, signal processing, controls, and medical robotics.
Proceedings
The proceedings shall be published shortly after the conference in IFAC-PapersOnLine