KEYNOTE SPEAKERS
MICNON2024 features the following keynote speakers:
- Prof. David Angeli
- Prof. Alexandre Bazanella
- Prof. Birgit Jacob
- Prof. Maryam Kamgarpour
- Prof. Sonia Martínez
- Prof. Necmiye Ozay
- Prof. Romain Postoyan
- Prof. Mario Sigalotti
Abstracts of plenary lectures
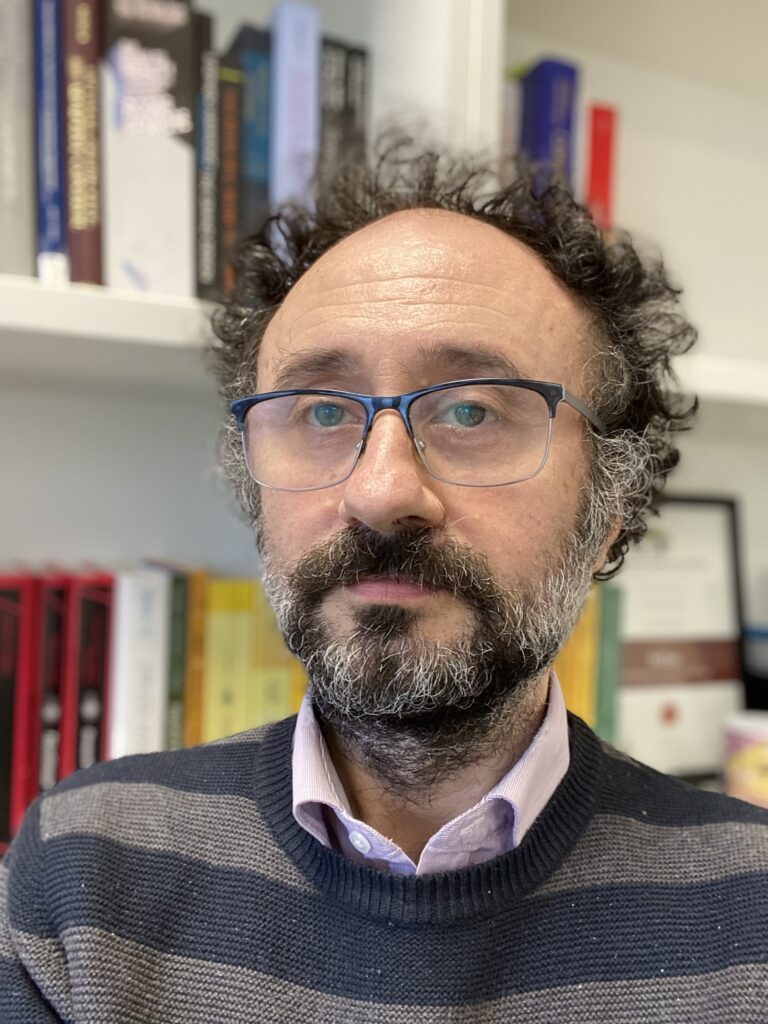
Prof. David Angeli, Imperial College, London, UK
David Angeli is currently a Professor in Nonlinear Network Dynamics at the Dept of Electrical and Electronic Engineering of Imperial College London after joining the group in 2008 as a Senior Lecturer. He is also an Associate Professor in the Dept of Information Engineering of University of Florence, Italy. He graduated in Computer Science from Florence University in 1996 and subsequently achieved his PhD in Control Theory from the same University (2000). He has held visiting positions at several institutions, including Rutgers University (New Jersey) and INRIA de Rocquencourt (France). He is a Fellow of the IEEE since 2015 and of the IET since 2018. He has authored more than 100 journal papers in the areas of stability of nonlinear systems, chemical reaction networks, model predictive control and smart grids.
A contraction theoretic approach for analysis and control of non-oscillatory systems
Non-oscillatory systems can be defined as systems whose solutions asymptotically approach the set of equilibria, be it a singleton or a set of higher cardinality. Standard contraction theory is only appropriate to deal with the case of a unique globally asymptotically stable equilibrium, whereas an interesting variant, originally proposed by Prof J Muldowney, allows to study them under relaxed technical assumptions. His elegant theory, recently recast under the name of 2-contraction (or k-contraction in the general case), is the focus of our talk. It is based on suitable generalization of the variational equation, which are formulated by taking into account the so called second additive compound of the system’s Jacobian, which allows to study how infinitesimal two-dimensional “area” perturbations propagate along the flow of the system. LMIs or Lyapunov functions can be set-up to test for contraction in this relaxed sense and interesting convergence result be achieved even for systems with multiple equilibria. We provide an introduction to the theory, its fundamental results when it comes to the study of the qualitative asymptotic behaviour of nonlinear systems, as well as applications in the area of chemical reaction networks, chaos control, and small-gain theorems under relaxed conditions.
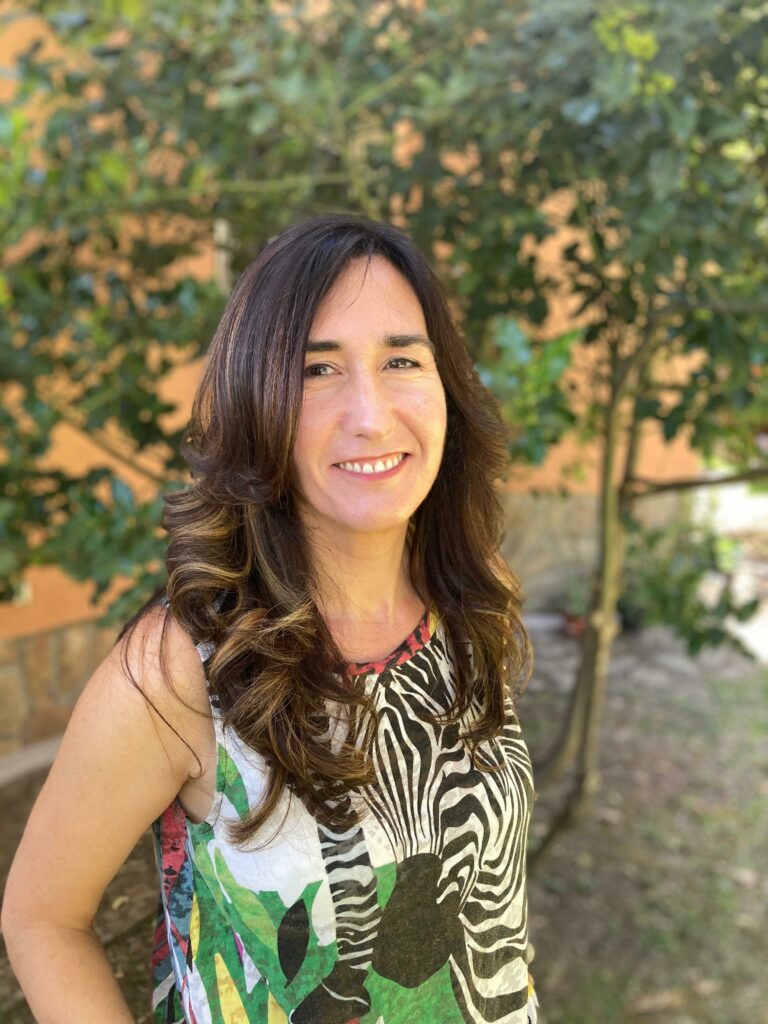
Prof. Sonia Martínez, University of California, San Diego, USA
Sonia Martínez is a Full Professor at the Department of Mechanical and Aerospace Engineering at the University of California, San Diego and a Jacobs Faculty Scholar. Prof. Martínez received her B.S. degree from the Universidad de Zaragoza, Spain in 1997, and her Ph.D. degree in Engineering Mathematics from the Universidad Carlos III de Madrid, Spain, in May 2002. Following a year as a Visiting Assistant Professor of Applied Mathematics at the Technical University of Catalonia, Spain, she obtained a Postdoctoral Fulbright Fellowship and held appointments at the Coordinated Science Laboratory of the University of Illinois, Urbana- Champaign during 2004, and at the Center for Control, Dynamical systems and Computation (CCDC) of the University of California, Santa Barbara during 2005. From January 2006 to June 2010, she was an Assistant Professor with the department of Mechanical and Aerospace Engineering at the University of California, San Diego. From July 2010 to June 2014, she was an Associate Professor with the department of Mechanical and Aerospace Engineering at the University of California, San Diego.
Measuring and enhancing network resilience: metrics, learning, and defense strategies
Resilience, understood as the ability of a network to carry out its goals under adversarial attacks and unexpected failures, is critical for autonomy. Despite important advances in the design of distributed coordination and decision-making algorithms, multi-agent networks have proven fragile to targeted attacks. Novel theories and tools are therefore needed to guarantee resiliency of these systems, being the development of notions and techniques that characterize network resilience critical. However, obtaining such characterizations is difficult as resilience and performance are a complex function of the network’s and adversary’s capabilities, knowledge, resources, and the network interconnection structure. At the same time, we also need novel design methodologies that can protect multi-agent networks and adaptively manage their interconnection over time to achieve performance guarantees. In this talk, we present our recent progress in these directions including algorithmic methods for metric computation, data-driven algorithms for multi-agent learning, and defense strategies.
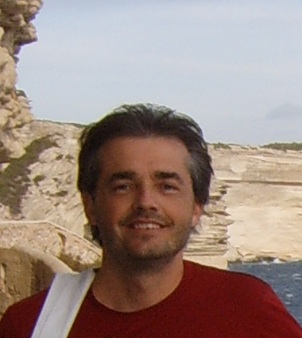
Prof. Alexandre Bazanella, Universidade Federal do Rio Grande do Sul in Porto Alegre-RS, BR
Alexandre Sanfelici Bazanella is a Full Professor with the Department of Automation and Energy of Universidade Federal do Rio Grande do Sul in Porto Alegre-RS, Brazil. He received his PhD degree in Electrical Engineering from Universidade Federal de Santa Catarina, Florian ́opolis-SC, Brazil, in 1997. He has held visiting professor appointments at Universidade Federal da Para ́ıba and at Universit ́e Catholique de Louvain, where he spent a sabbatical year in 2006. Dr. Bazanella is a member of the Brazilian Automation Society (SBA) and of the IEEE, both of which he has served in various roles: SBA vice- president, Editor of SBA’s Journal (Springer’s International Journal of Control Automation and Electrical Systems), associate editor of the IEEE Transactions on Control Systems Technology, member of the IEEE Board of Governors, and (currently) associate editor of the Control Systems magazine. In IFAC, he is a member of Technical Committees 1.1 and 2.2 and has served as General Chair of the 3rd Symposium on Systems Structure and Control. Dr. Bazanella has previously contributed to nonlinear systems theory, analysis and design of power systems controllers and electrical machine drives, and to the theory of system identification. For the past fifteen years his research interests and his contributions have been focused on the modeling and identification of multi- agent systems and on the theory and applications of data-driven control.
Nonlinear data-driven control
Following a very long and successful history of linear data-driven controllers, various approaches for nonlinear data-driven control have emerged in the past decade. These approaches blend various ultramodern concepts from systems sci- ence – the behavioral system description, kernel methods, deep learning – with traditional tools and ideas, like semi-definite programming, predictive control and model reference design. These data-driven design methodologies vary enor- mously in terms of their complexity, their algorithmic features and, perhaps most importantly, in terms of the quantity and quality of data they require. On the other hand, the theory is evolving rapidly, but meaningful application examples are still scarce, even at the simulation level. In this talk I’ll first give a bird’s eye view of these methodologies and their recent evolution. Then I’ll discuss, also by means of examples, the potentials and limitations of each ap- proach, providing insights into their most promising application fields and the theoretical and practical challenges lying ahead.
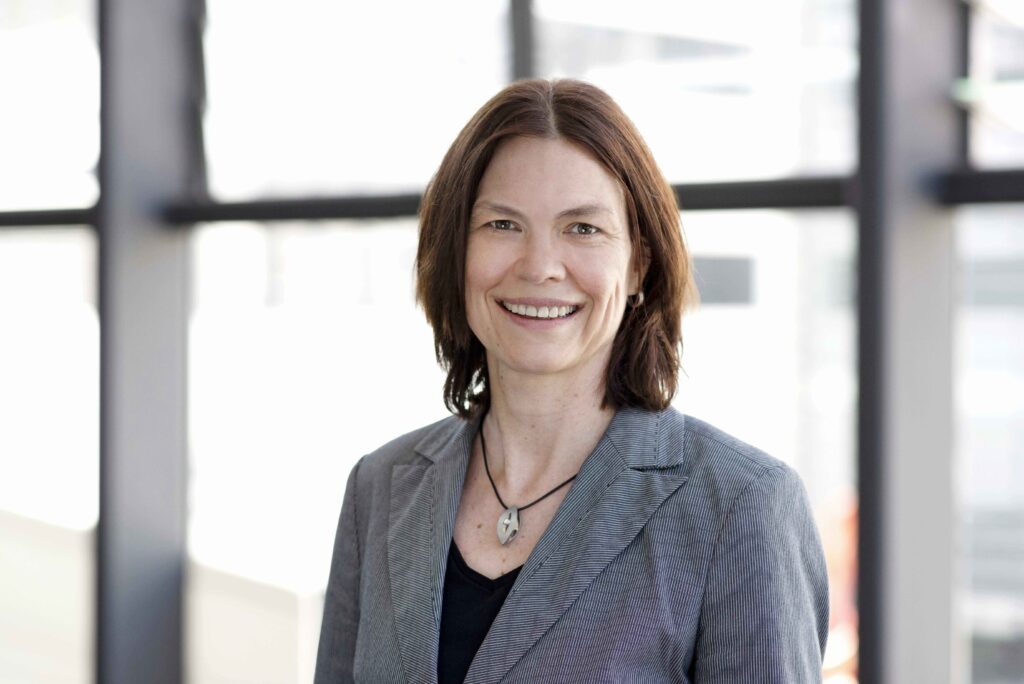
Prof. Birgit Jacob, Bergische Universität Wuppertal, GE
Prof. Birgit Jacob received the M.Sc. degree in mathematics from the University of Dortmund, Germany, in 1992 and the Ph.D. degree in mathematics from the University of Bremen, Germany, in 1995. She held postdoctoral and Professor positions at the Universities of Twente, Leeds and Paderborn, and at the TU Berlin, and TU Delft. Since 2010, she has been with the University of Wuppertal, Germany, where she is a Full Professor in analysis. From 2012-2016, she has been the Vice Dean of the Faculty of Mathematics and Natural Sciences. She is the coordinator of the EU MSCA Doctoral Network ModConflex. Her current research interests include the area of infinite-dimensional systems and operator theory, particularly well-posed linear systems and port-Hamiltonian systems.
Linear-Quadratic optimal control for a class of boundary controlled hyperbolic infinite-dimensional systems
In this talk the Linear-Quadratic optimal controls problem is investigated for a class of boundary controlled, boundary observed hyperbolic infinite-dimensional systems. The results are applied to two examples, a small network of three vibrating strings and a co-current heat-exchanger, for which boundary sensors and actuators are considered.
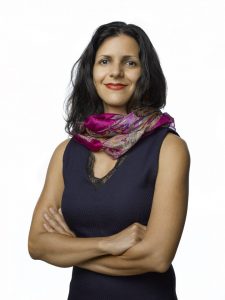
Prof. Maryam Kamgarpour, École Polytechnique Fédérale de Lausanne, CH
Maryam Kamgarpour is a professor in the School of Engineering of École Polytechnique Fédérale de Lausanne. Prior to EPFL, she served as a faculty at the University of British Columbia and at ETH Zürich. She holds a Doctor of Philosophy in Engineering from the University of California, Berkeley and a Bachelor of Applied Science from University of Waterloo, Canada. Her research is on stochastic control and multiagent learning and control. Her theoretical research is motivated by control problems arising in intelligent transportation systems, robotics, and power grid systems. She was awarded the European Union Starting Grant (2016-2021) to advance her research on control and game theory for integrating renewable energy into the power system. Her work on distributed control received the IEEE Transactions on Control of Network Systems Outstanding Paper Award (2022). She collaborated with NASA on safe and fuel-efficient aircraft trajectory design, and for this work received the NASA High Potential Individual Award (2010). She is passionate about understanding and addressing fundamental problems in control, and mentoring students to work with her on these problems. Her publications have contributed to theory of hybrid systems (reachability, safety and optimal control), distributed control (the role of information structure), game theory (learning equilibria under bandit feedback), mechanism design (coalition-proofness, price of anarchy), safe zeroth-order learning, inverse reinforcement learning and multiagent reinforcement learning.
Learning Nash equilibria with decoupled dynamics and zeroth-order information
Abstract: A rising challenge in control of large-scale control systems such as the energy and the transportation networks is to address autonomous decision making of interacting agents. Game theory provides a framework to model and analyse this class of problems. In several realistic applications, such as power markets, each player has partial information about the cost functions and actions of other players. Thus, a learning approach is needed to design optimal decisions for each player. This talk will spotlight recent developments on learning Nash equilibria in multiagent systems, where agents follow decoupled dynamics and use zeroth-order information of their cost functions. I will discuss conditions for convergence of such learning algorithms in static games, and their convergence rates. Building on this understanding, I will discuss some of the challenges in learning equilibria in dynamic games, present a few cases for which we have convergence guarantees, and conclude with highlighting some open directions.
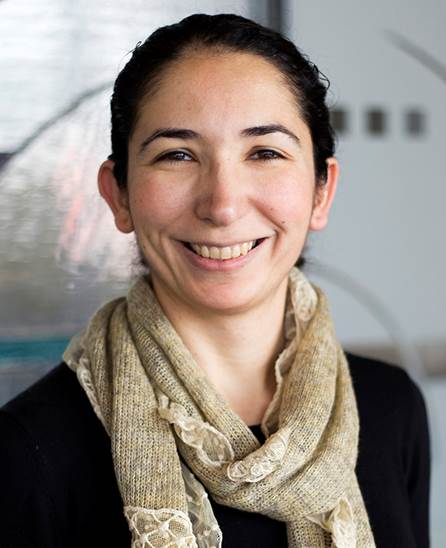
Prof. Necmiye Ozay, University of Michigan, USA
Necmiye Ozay is the Chen-Luan Family Faculty Development Professor of Electrical and Computer Engineering, and an associate professor of Electrical Engineering and Computer Science, and Robotics at the University of Michigan, Ann Arbor. She received her PhD in Electrical Engineering from Northeastern University in 2010. After a postdoctoral position at Caltech in Computing and Mathematical Sciences, she joined Michigan in 2013. Her research interests include dynamical systems, control, optimization, and formal methods with applications in learning-enabled cyber-physical systems, system identification, verification and validation, and safe autonomy. She received the 1938E Award and a Henry Russel Award from the University of Michigan for her contributions to teaching and research. She received five young investigator awards, including NSF CAREER Award. She is also the recipient of the 2021 Antonio Ruberti Young Researcher Prize from the IEEE Control Systems Society for her fundamental contributions to the control and identification of hybrid and cyber-physical systems.
Some fundamental limitations of learning for dynamics and control
Data-driven and learning-based methods have attracted considerable attention in recent years both for the analysis of dynamical systems and for control design. While there are many interesting and exciting results in this direction, our understanding of fundamental limitations of learning for control is lagging. This talk will focus on the question of when learning can be hard or impossible in the context of dynamical systems and control. In the first part of the talk, I will discuss a new observation on immersions and how it reveals some potential limitations in learning Koopman embeddings. In the second part of the talk, I will show what makes it hard to learn to stabilize linear systems from a sample-complexity perspective. While these results might seem negative, I will conclude the talk with some thoughts on how they can inspire interesting future directions.
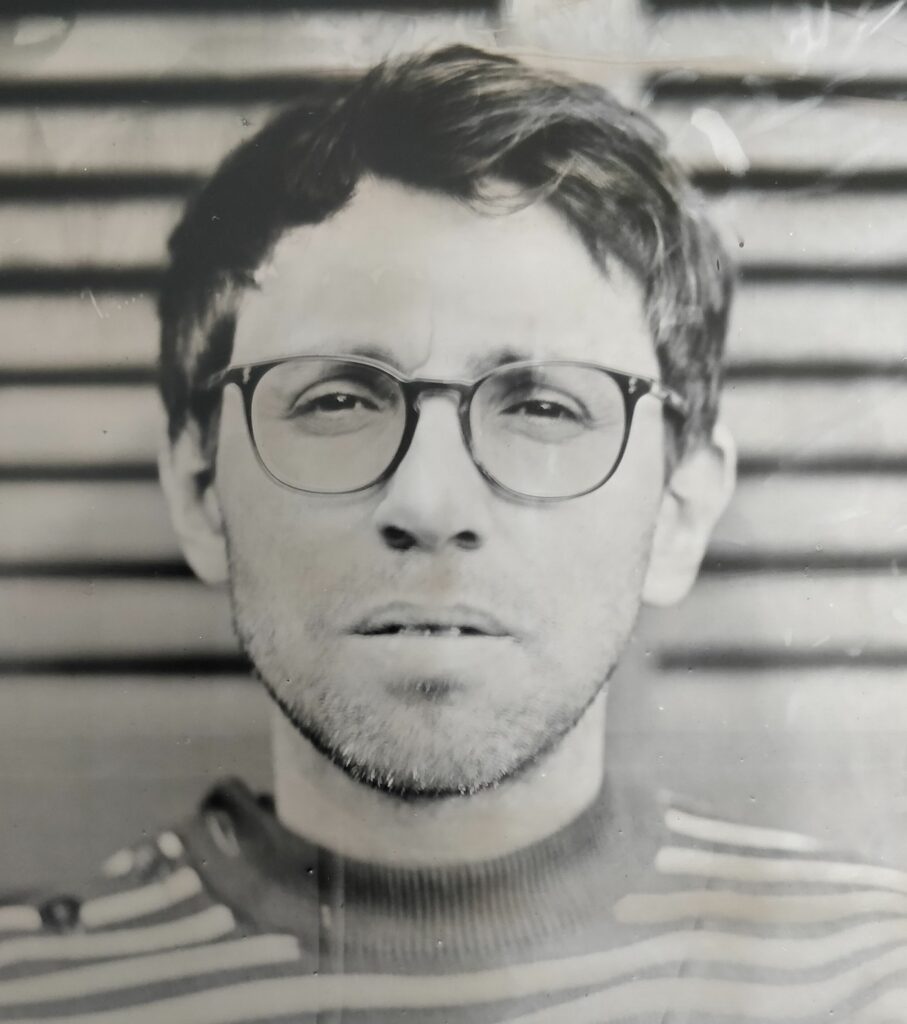
Prof. Romain Postoyan, CNRS, CRAN (FR)
Romain Postoyan received the “Ingénieur” degree in Electrical and Control Engineering from ENSEEIHT (France) in 2005. He obtained the M.Sc. by Research in Control Theory & Application from Coventry University (United Kingdom) in 2006 and the Ph.D. in Control Theory from Université Paris-Sud (France) in 2009. In 2010, he was a research assistant at the University of Melbourne (Australia). Since 2011, he is a CNRS researcher at CRAN (France). He serves/served as an associate editor for the journals: IEEE Transactions on Automatic Control, Automatica, IEEE Control Systems Letters, “Techniques de l’ingénieur” and IMA Journal of Mathematical Control and Information. His fields of interest include: Lyapunov stability, networked control systems, event-triggered control, hybrid systems, dynamic programming, nonlinear estimation, lithium-ion batteries.
When dynamic programming meets Lyapunov theory: robust stability and improved near-optimality guarantees
Dynamic programming (DP) plays a major role in various fields including reinforcement learning, operations research, computer sciences and of course control engineering. DP allows to solve general optimal control problems in terms of dynamical systems and cost functions. When exploiting DP in a control engineering context, it is often essential to endow the closed-loop system with stability guarantees. In this talk, we will present general conditions rooted in Lyapunov theory under which deterministic systems whose inputs are generated by a DP algorithm (like value iteration or policy iteration) exhibit stability properties. Interestingly, we will see that the presented Lyapunov-based conditions can be exploited to analyse the near-optimality properties of the algorithm used to generate the control inputs, thereby improving existi ng near-optimality bounds of the DP literature.
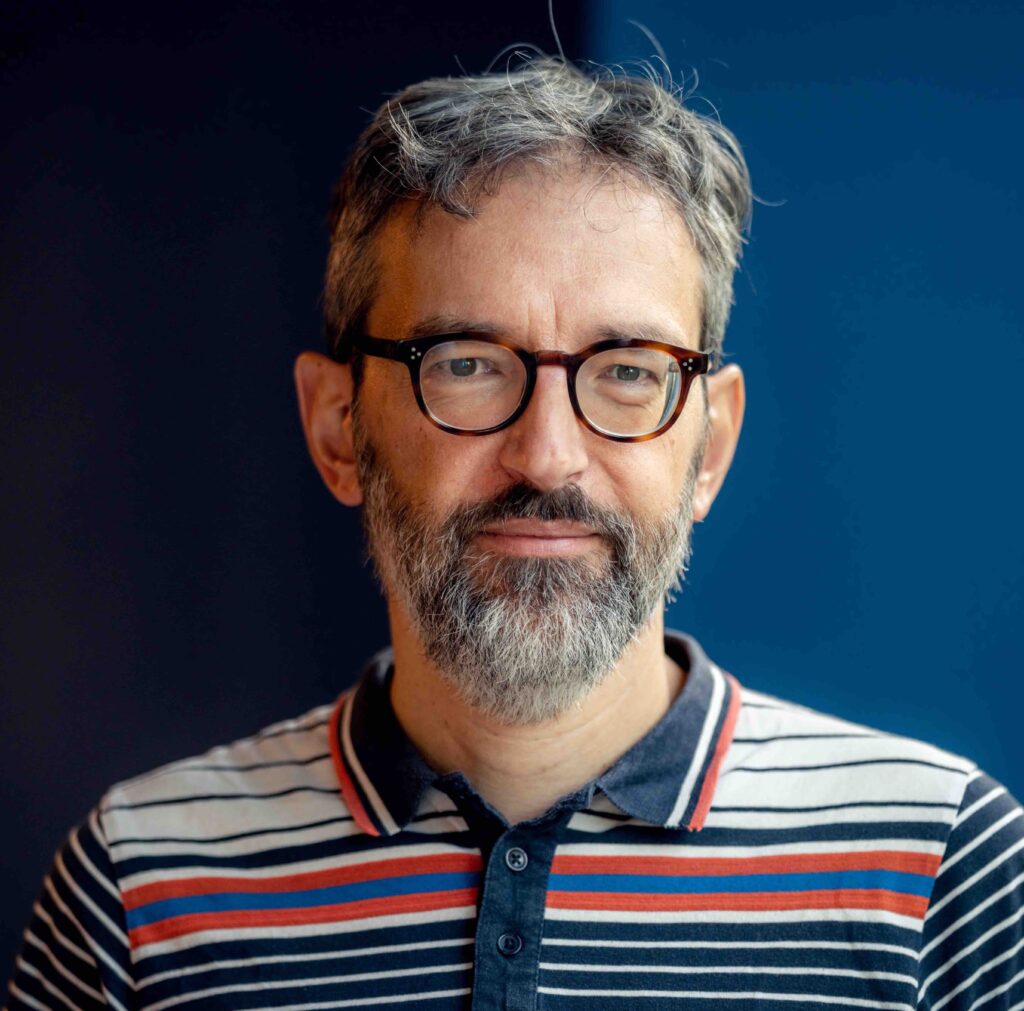
Prof. Mario Sigalotti, INRIA (FR)
Mario Sigalotti was born in Udine, Italy, in 1975. He has been undergraduate student in Mathematics at University of Trieste, Italy, and then Ph.D. student at SISSA, Trieste, Italy. He was Marie Curie Post-doc Fellow at Inria Sophia Antipolis, France. Since 2005, he is permanent research at Inria, first in Nancy and then in Paris, France. He is currently member of the Laboratoire Jacques-Louis Lions, Sorbonne Université, Paris, where he heads the Inria team CAGE. His research interests are in the area of geometric control theory and include control of quantum systems, optimal control, and stability of switched systems.
Some features in the controllability analysis of closed quantum systems
We present some of the peculiarities showing up while studying the controllability of closed quantum systems. The latter are bilinear systems with unitary admissible flows, and may occur both in finite-dimensional and infinite-dimensional Hilbert spaces. We discuss the relation between approximate and exact controllability for such systems (with some extensions to general bilinear systems). We then consider both small-time controllability (and obstructions to it) and long-time controllability by quasi-static controls. We finally discuss how the latter play an important role in the analysis of ensemble controllability.